by Satalia Team
10 April, 2025
How good is AI at understanding context behind data?
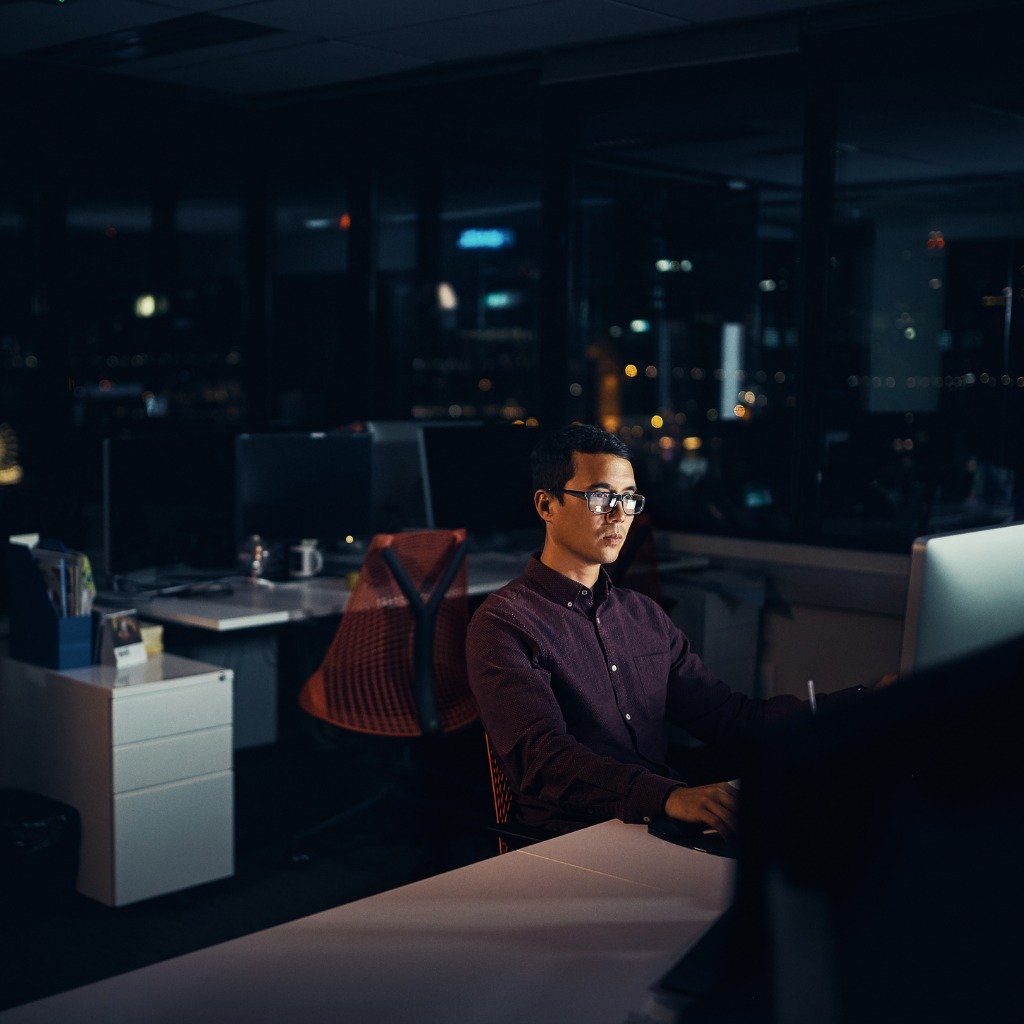
Just because an AI system gets better at making decisions with data, doesn’t mean it understands the problem. Without context, it’s not intelligent, it’s just efficient. And doing the same thing over and over without questioning it? That’s not intelligence. That’s automation. Or, depending on who you ask…madness….
What does “understanding context” even mean in AI terms?
At its core, understanding context means interpreting the relationships between pieces of information to grasp the bigger picture. For people, this comes naturally. We rely on intuition, experience, and nuanced reasoning to connect dots and infer meaning. AI, however, approaches context differently. It relies on data patterns, mathematical models, and training to identify correlations and make predictions.
But does that mean AI truly understands the data it’s working with? Definitely not…
Can AI identify “why” something happens?
AI systems can process vast amounts of data and recognise patterns across dimensions that humans cannot. For example, machine learning algorithms can detect a customer’s purchasing behaviour and predict future buying trends based on seemingly unrelated variables. But this process is driven by correlations, not comprehension. AI doesn’t inherently “know” why one factor influences another. It simply identifies patterns from the data provided.
AI is excellent at answering “what” questions: What is likely to happen next? What patterns exist in this data? What do customers want? However, it often struggles with the “why?” That’s because contextual understanding requires more than pattern recognition. It needs reasoning and a sense of cause-and-effect relationships.
To infer “why,” humans often bring domain expertise, cultural awareness, and emotional intelligence — qualities that current AI systems lack. While AI might identify that a trend exists, it’s humans who unpack the reasoning behind it.
How does AI navigate complexity beyond human capability?
Humans excel at interpreting up to four dimensions simultaneously, like height, width, depth, and time. AI can handle thousands of dimensions, uncovering patterns and relationships far beyond human capacity.
For example, in workforce allocation, assigning 60 employees to 60 projects creates more possible combinations than the number of atoms in the observable universe. AI excels at analysing these combinations, satisfying constraints, and finding optimal solutions. It simply processes the data it’s given, adhering to predefined rules and objectives. And this is where AI really earns its keep — solving mind-bending problems at speeds no human could match. It’s not thinking, it’s calculating. And when the challenge is “allocate 60 people to 60 projects without breaking the universe,” that’s exactly what you want.
The limitations arise when context isn’t explicitly included in the data. AI might not know, for example, that a particular employee shouldn’t be assigned to a project due to sensitive religious or personal reasons — details that aren’t stored in the dataset for privacy reasons. This is where human input becomes essential, providing the nuance and ethical judgement that AI can’t.
Does that mean AI has absolutely no strengths in contextual analysis?
While AI might struggle with reasoning and interpretation, it has specific strengths in contextual analysis that are worth noting. For example, machine learning models excel at identifying correlations that humans might miss. In industries like healthcare, AI can detect patterns in medical data, linking symptoms to conditions in ways that improve diagnostics and treatments. In marketing, AI analyses customer data to personalise recommendations, creating context-driven campaigns that resonate with audiences.
These strengths highlight AI’s potential as a powerful analytical tool. When used responsibly, AI can uncover insights that enhance decision-making, even if it doesn’t fully “understand” the context behind those insights.
So how can humans and AI complement each other?
Rather than relying on AI to fully comprehend data, organisations can use it as a tool to augment human decision-making. AI handles the heavy lifting, crunching numbers and running simulations, while humans provide the intuitive context that AI lacks.
In that example of the workforce allocation project, planners define the constraints. The magic then happens when machines handle the grind, and humans bring in their judgement. AI doesn’t care if someone’s off on parental leave, crumbles in early meetings, or has a complicated working relationship with a particular client. But people do — and those things matter.
AI uses these constraints to generate multiple scenarios, and humans evaluate which scenario best suits the situation based on context. That’s why the best decisions aren’t made by AI or humans alone, but by both, working together.
Does explainability bridge the gap?
One way to possibly address some of AI’s shortcomings in understanding context is by making its decisions more explainable. Explainability allows organisations to see how AI arrived at a particular outcome, revealing the patterns and correlations it identified. This transparency enables humans to identify potential biases, refine models, and better trust the system. It’s also how we stop AI from becoming a mysterious oracle in the corner. If it’s going to make decisions that affect people’s lives, it has to show its workings. No magic tricks. No black boxes. Just clear logic real people can interrogate.
At Satalia, for example, explainability is a core principle in our enterprise AI development. The goal isn’t just to create powerful algorithms but to ensure decisions can be understood and trusted. If AI recommends a particular course of action, decision-makers should be able to see the reasoning behind it, empowering them to intervene when needed, and to explain them to clients, customers, auditors, etc.
Explainability doesn’t give AI contextual understanding, but it does make its processes more accessible and understandable to people. By showing the logic behind AI’s decisions, explainability allows for greater collaboration between humans and machines, ensuring decisions align with organisational goals and values.
How do we use AI responsibly when context is critical?
When context matters, using AI responsibly means recognising its limitations and designing systems that work in harmony with human expertise. Organisations must ensure their AI systems are built with transparency, adaptability, and accountability in mind. This involves:
Defining clear objectives
Ensuring AI systems are designed to achieve specific goals without overreaching or causing unintended consequences.
Incorporating explainability
Making AI decisions transparent so humans can understand and refine the process.
Involving humans in the loop
Keeping people at the centre of decision-making, particularly in areas where cultural or ethical context is critical.
Adapting to new challenges
Building AI systems that can evolve as new data and contexts emerge, ensuring they remain relevant and effective.
Responsible AI use isn’t just about building better systems. It’s about fostering collaboration between humans and machines to solve problems more effectively.
Will future AIs ever understand context?
The question of whether AI can ever achieve true contextual understanding is tied to the evolution of its capabilities. The current wave of generative AI systems, like ChatGPT, are limited to recognising patterns and optimising objectives within the constraints of their training data. However, emerging technologies like neuromorphic computing could bring us closer to AI that mimics human-like reasoning.
Neuromorphic computing is designed to replicate the structure and function of the human brain, using artificial neurons to process information more intuitively. These systems have the potential to learn and adapt in real-time, addressing some of the challenges AI faces with contextual understanding. For instance, a neuromorphic system could not only identify that an ad campaign is performing well but also adapt its approach based on real-time feedback, making smarter decisions over time.
While neuromorphic computing represents a significant leap forward, it’s important to recognise that AI will never fully replicate human intuition. Context is messy. It lives in tone, timing, culture, history — the kind of understanding you pick up over years of being alive, not milliseconds of processing. AI can become better at mimicking these qualities, but its role will always be to complement human intelligence, not replace it.
So do we really need AI to understand context?
We don’t need AI to think like us. We need it to be great at what it does so we can be great at what we do. Let it sweat the small stuff, while we apply the big picture considerations.
AI is incredibly powerful at recognising patterns, optimising decisions, and processing complex data at scales humans can’t match. But when it comes to understanding the “why” behind data, it falls short.
Instead of striving for AI to fully understand context, we should focus on leveraging its strengths while addressing its limitations. By combining AI’s analytical capabilities with human judgement, we can achieve outcomes that are both effective and contextually meaningful. The future of AI isn’t about replacing humans but empowering them to make better decisions in an increasingly complex world.
Speak to an expert Satalia advisor today about how AI can transform your business decision-making.
Stay in the know
Join our community now for the latest industry news, trends, valuable insights, and updates on our products and services.